Unlocking the Power of **Machine Learning Labeling Tools** for Your Business
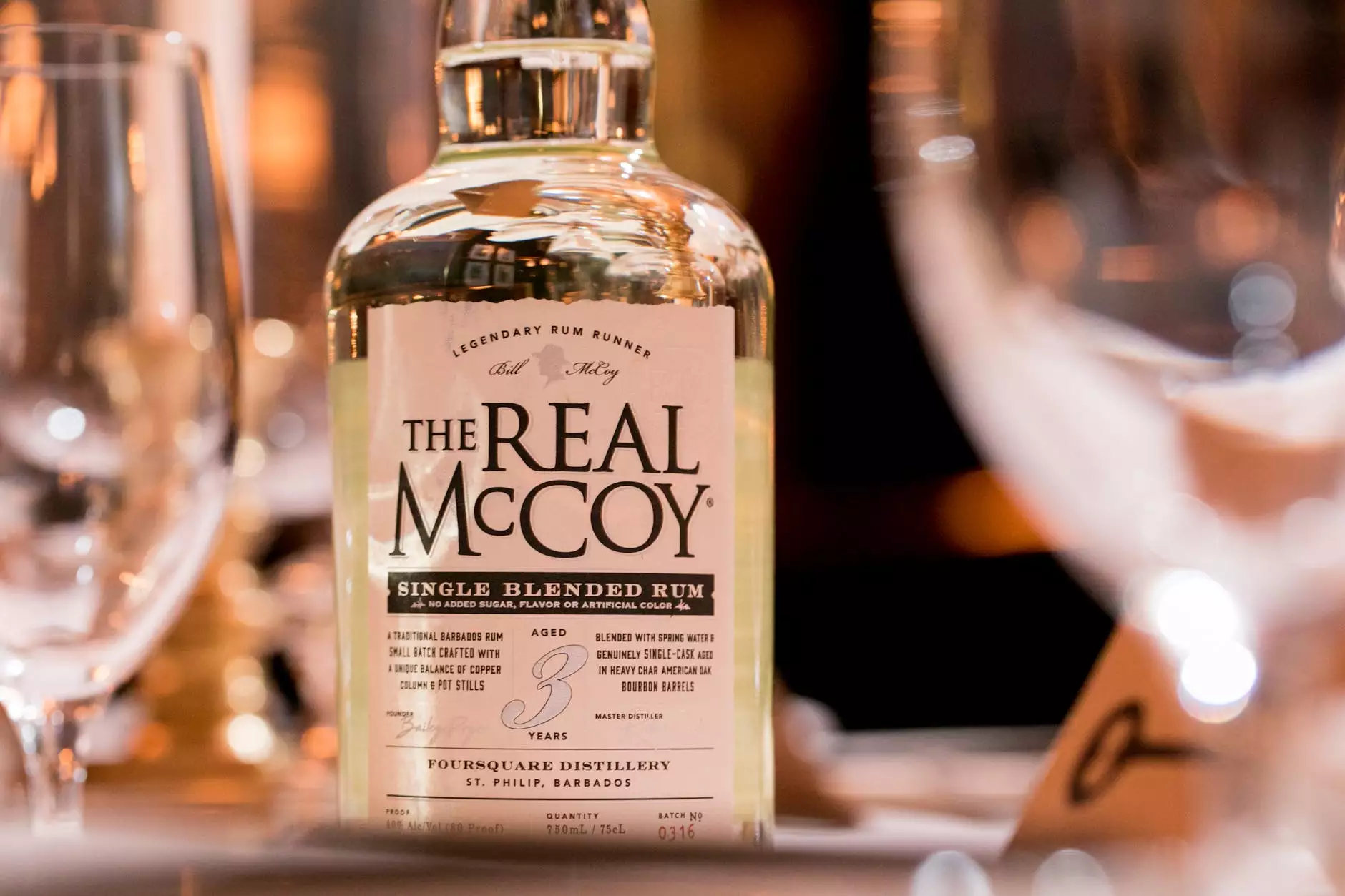
In the evolving landscape of artificial intelligence and machine learning, the significance of data cannot be overstated. As businesses strive to harness the power of AI, the process of data annotation becomes a critical factor in determining the success of machine learning projects. One of the pivotal components in this workflow is the use of machine learning labeling tools that facilitate accurate and efficient data labeling.
Understanding the Concept of Data Annotation
Data annotation is the process of labeling data to be used in machine learning algorithms. This data can come in various forms, including images, text, video, and audio. Annotations provide the necessary context for the algorithms to learn and make decisions. Here are key aspects to consider:
- Types of Data: Data can be structured or unstructured. Structured data includes numeric inputs, while unstructured data encompasses images and text.
- Annotation Techniques: Techniques can range from simple tagging to complex object detection and semantic segmentation.
- Quality of Data: The quality and accuracy of annotations directly influence the performance of machine learning models.
The Role of Machine Learning Labeling Tools
Machine learning labeling tools are designed to streamline the data annotation process. By providing intuitive interfaces and advanced features, these tools allow users to efficiently annotate large datasets. Here’s how they can benefit your business:
1. Increased Efficiency
Manual annotation can be a time-consuming process. With the right machine learning labeling tool, businesses can automate many aspects of data labeling. This efficiency translates into faster project deliveries and the ability to work with larger datasets. For instance:
- Batch Processing: Tools that support batch processing allow annotators to label multiple items simultaneously, significantly reducing time spent.
- Collaborative Features: Many platforms enable team collaboration, meaning multiple users can work together in real-time, ensuring consistency and reducing bottlenecks.
- Custom Workflows: Tailor workflows to your unique needs, simplifying the annotation process and making it adaptable to different projects.
2. Enhanced Accuracy
Accuracy is paramount in data annotation. Poorly annotated data can lead to flawed models and unwanted biases. Machine learning labeling tools come with features that enhance the accuracy of annotations:
- Quality Control Mechanisms: Built-in quality control checks can help ensure that each annotation meets specified standards.
- AI-Assisted Labeling: AI-driven suggestions can pre-label data, allowing human annotators to focus on corrections rather than starting from scratch.
- Annotation Templates: Predefined templates make it easier to maintain consistency across different projects and datasets.
3. Cost-Effectiveness
Investing in machine learning labeling tools can significantly reduce the cost associated with manual labeling. While there is an initial investment, the long-term savings can be dramatic. Consider the following:
- Reduced Labor Costs: Automating parts of the annotation process minimizes the need for a large team of data annotators.
- Faster Time-to-Market: Efficiency improvements lead to quicker project completions, allowing businesses to leverage AI solutions sooner.
- Scalability: Handle increased workloads without proportionally increasing costs by utilizing powerful labeling tools.
Key Features to Look for in a Machine Learning Labeling Tool
When selecting a machine learning labeling tool, businesses should consider various features to ensure they choose the right platform for their needs:
1. User-Friendly Interface
An intuitive interface is crucial for maximizing productivity. A good tool should enable users to quickly learn the system, thus reducing onboarding time for new team members.
2. Versatility
Choose a tool that can handle a variety of data types and annotation tasks, such as image segmentation, text labeling, and audio transcription. Versatility ensures that one tool can meet multiple project needs.
3. Integration Capabilities
The ability to connect with existing software and frameworks can enhance workflow efficiency. Look for tools that offer API access and can integrate with data storage solutions, project management software, and other necessary technologies.
4. Support and Documentation
A robust support system, including readily available documentation, tutorials, and customer service, can greatly improve user experience and help teams overcome challenges quickly.
How KeyLabs.ai Excels in Data Annotation Solutions
One of the leading providers of machine learning labeling tools is KeyLabs.ai. Their data annotation platform stands out in several ways:
1. Advanced AI-Powered Labeling
KeyLabs.ai utilizes cutting-edge technology that brings AI assistance to data labeling, which significantly reduces the time required for manual intervention. This allows for a hybrid approach where machines handle repetitive tasks while human annotators focus on complex cases, leading to improved accuracy.
2. Focus on Quality Assurance
The platform prioritizes quality by implementing numerous control mechanisms throughout the annotation process. Teams can conduct regular audits and quality assessments, ensuring every labeled dataset is reliable and trustworthy.
3. Tailored Solutions
KeyLabs.ai understands that each business has unique challenges. Their flexible configuration options allow clients to customize the labeling tool to fit their specific requirements, ensuring optimal performance and efficiency.
4. Extensive Support and Training
With dedicated support and extensive training resources, KeyLabs.ai helps organizations maximize the effectiveness of their data annotation efforts, ensuring that teams are well-equipped to use the tools available at their disposal.
Case Studies: Success Stories with Machine Learning Labeling Tools
Real-world examples illustrate the benefits of using machine learning labeling tools in various sectors:
1. Automotive Industry
In the automotive sector, companies have utilized labeling tools for the development of autonomous vehicles. Accurate labeling of images captured by cameras is essential for object detection algorithms. By leveraging automated labeling solutions, automakers have reduced the time needed to prepare datasets while increasing the quality of annotations.
2. Healthcare Sector
In healthcare, data annotation is critical for the development of machine learning models used in diagnostics. By using KeyLabs.ai, hospitals and healthcare providers have successfully annotated medical images, enhancing the capabilities of AI systems used for early disease detection. The accuracy and speed improvements have been instrumental in advancing patient outcomes.
3. Retail and E-commerce
In the retail industry, accurate data labeling has been vital for personalized recommendations. Retailers have implemented machine learning labeling tools to classify and tag product images, resulting in higher conversion rates and a better customer experience. The savings generated from operational efficiencies have allowed companies to reinvest in customer-centric initiatives.
Best Practices for Efficient Data Annotation
To maximize the benefits of machine learning labeling tools, businesses should adopt best practices for data annotation. These include:
1. Define Clear Guidelines
Establish clear annotation guidelines to ensure consistency across datasets. This will help annotators understand the objectives and maintain quality throughout the labeling process.
2. Continuous Training and Feedback
Provide ongoing training for your annotation team, and incorporate feedback mechanisms to identify areas for improvement. This promotes a culture of quality and excellence.
3. Monitor and Evaluate Performance
Regularly assess performance metrics to gauge the efficiency and quality of the annotation efforts. Utilize these insights to make data-driven decisions that enhance productivity.
The Future of Data Annotation with Machine Learning Labeling Tools
As artificial intelligence continues to advance, the need for effective data annotation will only grow. Organizations that invest in robust machine learning labeling tools will be well-positioned to harness the evolving capabilities of AI technologies. With improvements in automation, accuracy, and efficiency, these tools will play a critical role in shaping the future of data-driven innovation.
Conclusion
In conclusion, as businesses embark on their journeys into the world of AI and machine learning, the significance of machine learning labeling tools cannot be emphasized enough. By enhancing the efficiency, accuracy, and overall quality of data annotation, these tools empower organizations to make data-backed decisions with confidence. With KeyLabs.ai, your data annotation strategies can reach new heights, driving growth and competitive advantage in a rapidly evolving digital landscape.